Abstract
In the world, environmental priorities are becoming increasingly important, especially important for fragile ecosystems such as the Arctic. These territories require close attention, due to the increasing frequency of man-made accidents, the bottling of oil products. Tank farms, which in many ways have already exhausted their design resource, are becoming a zone of increased attention, but are still in operation. In order to reduce man-made risks caused by depressurization of tanks with oil products, it is necessary to organize continuous monitoring and forecasting of risks, assess possible consequences, organize preventive measures, and develop response plans in the event of emergencies. It is necessary to create complex security systems at an accelerated pace to prevent and eliminate the consequences of natural and man-made emergencies in the Arctic zone. The work solved the problem of modelling the development of events during emergency bottling of oil products at a tank farm in the Arctic zone of the Krasnoyarsk Territory. The assessment of the area of bottling of oil products into the reservoir has been carried out. Modelling of emergency situations was carried out using the “Toxy+” risk software product, and the neural network forecasting methodology using the neural network simulator “NeuroPro”, developed at the Institute of Computational Modelling of the Federal Research Center of the KSC SB RAS to estimate the bottling area until the moment of localization. Comparison of the analytical method for determining the area of the oil spill in a reservoir and the neural network method is made.
Keywords: Toxy+, NeuroPro, neural network method, arctic enterprises, risk
Introduction
The Arctic is a territory where the geopolitical and strategic interests of the developed economies of the world clash. The economic value of this territory is difficult to assess, since every year, with the development of geological exploration technologies, more and more new deposits of oil, gas, minerals, coal and brown coal, gold and platinoids and rare earth elements are being discovered. Projects for the development of these minerals are key for the development of the economy of the regions and the Russian Federation as a whole.
On the territory of the Krasnoyarsk Territory in the Arctic zone, the Taimyr-Turukhansk support development zone is being created. When creating support zones for development, especially when implementing major economic and infrastructure projects in areas of industrial development and areas of active development, that is, where infrastructure (transport, energy and social frameworks will be created at a faster pace), a sharp increase in the number of people is expected, which in its own turn leads to the need to provide equipment with fuels and lubricants and an increase in stationary tanks for storing fuel, since due to the specifics of the transport infrastructure and meteorological features of the Artik, the task of uninterrupted and continuous supply of petroleum products is difficult. In this regard, all deliveries are made as part of the northern delivery from spring to autumn, and fuel is stored in tanks, which have already largely exhausted their resource and can carry a potential threat of an emergency caused by depressurization of these tanks.
Problem Statement
Within the framework of the integrated development of the Arctic, the city of the port of Dudinka, which is an intermediate point of the northern sea route, is of the greatest importance. On the territory of the port, located on the right bank of the Yenisei River, there is: an onshore tank farm where there is a significant number of tanks with oil products; a pumping station for internal pumping; onshore process pipelines, diesel power plants; the operation of which in extreme weather conditions increases the risk of emergencies associated with bottling petroleum products.
In the vicinity of the above-ground reservoir park, there are lakes located at a distance of 30 m (with a total area of over 18 thousand m2); streams flowing into the Yenisei River with a length of about 500 m to the confluence with the Yenisei River. During the flood period, the width of the streams can reach 1.5 m, and the speed of the current up to 2.5 m/s.
Despite all the measures taken in the field of industrial safety, accidents continue to occur and their consequences bring significant harm to the environment and human health. In 2020, three major accidents were recorded in the Arctic zone of the Krasnoyarsk Territory, as a result of which oil products got into the soil and water bodies, causing irreparable damage to the environment.
The analysis of emergencies at fuel oil storage facilities over the past 10 years allows us to conclude that the overwhelming number of accidents is associated primarily with the aging of the tank farm, which complicates the problem of monitoring the state of the tank farm and predicting possible consequences.
The problem of creating an effective risk management system for technogenic systems was considered in the works of the authors (Medvedeva & Solovieva, 2016; Mikheeva & Ayusheeva, 2014; Moskvichev & Nicheporchuk, 2018; Moskvichev et al., 2017; Potravny & Ngo, 2018). The significance of the issues of forecasting emergency situations was raised in the works of a number of researchers such as Korovin (1969), Grebnev et al. (2019), Grebnev and Moskalev (2020), Dorrer (2008), Volchek (2010), Chuchueva (2010). The problems of regulating the use of polluted lands and water bodies were considered in the works of the authors (Cappuyns, 2013; Carlon et al., 2007; Fentiman & Zabbey, 2015). Carrying out effective preventive measures is impossible without organizing the monitoring and forecasting process. Risk forecasting methods based on machine learning have become widespread and have found application in applied and fundamental research (Gorban et al., 2002; Grebnev & Yarovoy, 2018; Martyinov, 2011).
Research Questions
The work solved the problem of modelling the development of events during emergency bottling of oil products at a tank farm in the Arctic zone of the Krasnoyarsk Territory.
Purpose of the Study
In this work, the goal was to develop a model for assessing the impact of the risks of an emergency situation caused by the bottling of oil products. The adequacy of the model correlated with the incident at the oil depot in the area of the settlement of Dudinka located beyond the Arctic Circle.
Research Methods
Simulation of an emergency situation at a tank farm was carried out using the TOXI + Risk software product (Toxi Industrial Safety Software, 2021) and the neuroimitator NeuroPro 0.25 developed at the Federal Research Center of the KSC SB RAS (NeuroPro, 2021). The data set for risk assessment and modeling was obtained from the Main Directorate of the Ministry of Emergency Situations of Russia for the Krasnoyarsk Territory, the agency for civil defense, emergency situations and fire safety of the Krasnoyarsk Territory on the basis of emergencies that occurred in 2020.
The initial data for the calculations were the following indicators:
- density of oil poil = 850 km/m3;
- coefficient of spread of petroleum products on concrete pavement fp = 30 m-1;
- the temperature of the oil product obtained by calculation tp = 39 °C;
- flame spread concentration limit φf = 0.9%;
- molecular weight of oil M = 50 kg/mol;
- molar volume of steam V0 = 22.41 m3/kmol;
- free fall acceleration g = 9.8 m/s2;
- atmospheric pressure p0 = 101.325 kPa;
- VST (vertical steel tank) height, Нр = 10.92 meters;
- VST (vertical steel tank) diameter, Dp = 40 meters;
The work considered the following emergency scenarios:
- Destruction of an above-ground vertical steel tank.
- Destruction of the onshore process pipeline.
- Overflow of a vertical steel ground tank.
- Destruction of the onshore process pipeline in the area of possible impact on the lake.
- Destruction of the onshore process pipeline in the area of possible impact on the stream.
- Destruction of the onshore process pipeline in the area of possible impact on the Yenisei River.
The following formula is used to estimate the area of spilled oil products in a free spill on land (Tsalikov, 2009a):
(1)
where is the area of spilled oil products, f is the spill coefficient, which is taken: when light oil products are spilled onto the surface of concrete or asphalt - 150 m-1 and when light oil products are spilled onto the ground - 20 m-1.
Voil - volume of spilled oil products, m3.
Layer thickness of spilled oil products on land in flanging/embankment (m):
(2)
Sо - equal to the flanged / bunded area minus the area of technological elements (tanks, buildings, etc.).
Depending on the location of the source of the oil spill on the ground, the oil spill can take different geometric shapes.
When oil products are spilled in a lowland or on a flat surface, the spill shape is a circle with a radius (m):
(3)
When an oil spill occurs in an area with various restrictions (embankment, flanging, processing equipment, etc.), the shape of the oil product spill is characterized by the geometric characteristics of the limited areas.
The maximum predicted oil spill area on the water surface is approximated by the formula (Gosgortekhnadzor of Russia, 2002):
m2 (4)
If the water surface area is less than the maximum predicted oil spill area on the water surface, calculated using the above formula, then the maximum predicted oil spill area on the water surface is assumed to be equal to the surface area of the water surface.
When entering stagnant water bodies, oil products begin to concentrate on the water surface and their spreading over the water surface is limited by the banks.
When entering watercourses, oil products begin to concentrate on the water surface. Its spreading over the water surface of water areas is limited by the shores, and spreading occurs downstream.
Taking into account the uneven distribution of river flow velocities along their depth, the time and extent of the spread of the oil slick is calculated based on the wind speed and the maximum flow speed. When a slick of a spilled oil product spreads along the course of streams (due to the shallow depth), the speed of their flow can be considered constant. When considering the scenario of an accident of destruction of the onshore process pipeline in the area of possible impact on the stream. the entire water area of the stream will be contaminated in less than 5 minutes and spilled oil products will begin to flow into the water area of the Yenisei River.
Then the maximum distance in meters to which a slick of oil products will spread is calculated by the formula (Rostekhnadzor, 2015):
(5)
where: - maximum flow rate (m/s):
(6)
where: is the average flow rate of the Yenisei River at the point of confluence of the stream and downstream, m/s (based on the worst conditions: the average speed of the current during the spring flood (April-May) at the point of confluence of the stream and downstream is - 1.50 m/s); - wind speed, m/s (based on the worst conditions: the average wind speed during a spring flood (April-May) in the area of possible ES (N) is 6 m/s, and the wind direction coincides with the direction of the Yenisei River); is the time after the start of the accident (discharge of oil products into the water body), in seconds.
The analytical solution to the problem of the distribution of oil products in the reservoir was compared with the solution to the problem of the distribution of oil products produced by means of using artificial neural networks, trained on the analysed data of the bottling of oil products in Norilsk in 2020.
To solve the problem of modelling the ingress of oil products into the Yenisei River, we used multilayer neural networks with a sigmoid activation function of the form:
(7)
where: is the characteristic of the neuron;is a set of input parameters.
The neural network training algorithm was reduced to training and testing a network with predetermined characteristics. The dimensions of the area for bottling oil products in a reservoir depend on many factors that should be taken into account when modeling and compiling feature vectors:
- 1.the volume of oil that has entered the reservoir;
- protective structures and their type on the path of distribution of oil products;
- terrain relief;
- the level of watering of the area;
- the presence of vegetation: woody, shrub, herbaceous;
- ambient temperature;
- time of spill containment;
- the amount of precipitation;
- mass of oil dispersed in water, tons;
- wind speed, m/s;
- speed of water flow, m/s;
- type of oil product;
- wind direction.
The input parameters were selected empirically. The dimension of the vectors of the features noted above was established empirically, was thirteen. In the calculation, a multilayer neural network with 12 layers and the number of neurons in hidden layers equal to 204 was used, which shows good results in practice. The percentage of reliability of the results obtained was in the range of 71-78%.
Modeling scenarios of oil product combustion (spill, fire-flash, explosion) in accordance with the Methodology for determining the calculated values of fire risk at production facilities (Tsalikov, 2009b), using the TOXI + Risk software product.
Findings
In accordance with the methodology described in Section 2 of the work, using the TOXI + Risk software product, the following results of modelling the risk of an accident at a tank farm were obtained, Table 1.
The first group of scenarios includes modelling of emergency situations associated with the bottling of oil products on land.
As follows from the above data, the largest area of oil spills into the ground occurs when a ground vertical steel tank is depressurized. This is primarily due to the volume of stored petroleum products, which, as a result of destruction, will be completely emptied.
Consider further the scenarios of bottling oil products entering the reservoir, Table 2.
Figure 1 shows the zone of maximum possible contamination by spilled oil products (with full containment in 4 hours)
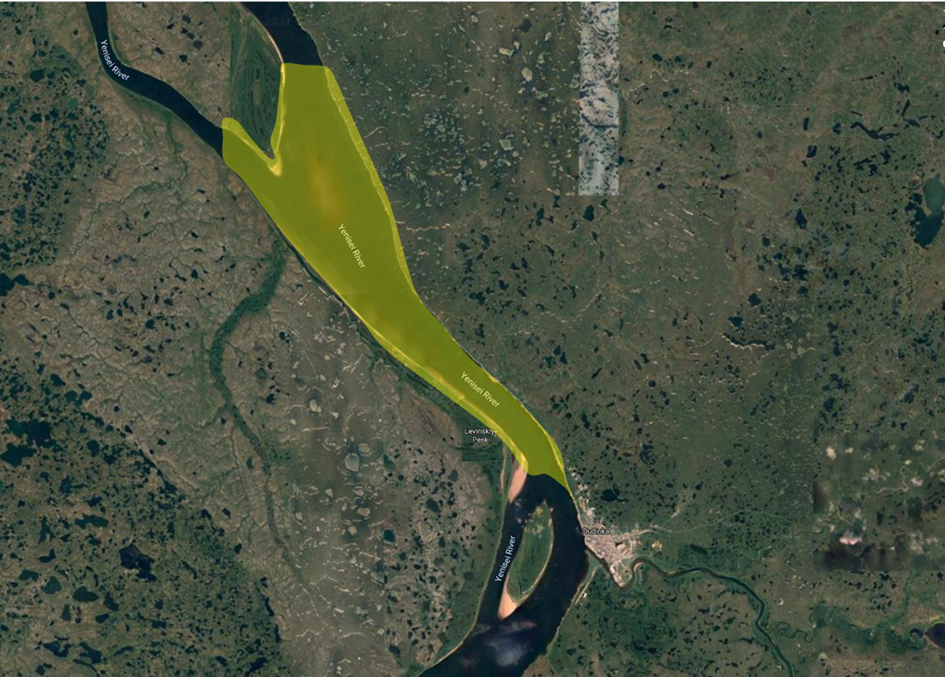
As a result of the ingress of oil products into the reservoir, toxic contamination of the environment will occur (soil pollution of the coastal strip), the water area of the Yenisei River, atmospheric air by the products of evaporation of oil products).
Let us consider the situational models that define the boundaries of oil spill zones, taking into account the results of assessing the risk of oil spills.
In the event of a spill of oil products on land, into a stagnant body of water, the formation of secondary consequences is possible, in the case of a spill of oil products in the water area of the river, secondary consequences are unlikely and are not considered.
The boundaries of the areas for bottling oil products in the event of and secondary consequences of the accident are shown in Table 3.
When oil products are spilled on areas with various restrictions (embankment, flanging, processing equipment, etc.), the shape of the oil product spill is characterized by the geometric characteristics of the limited areas.
Conclusion
In this work, the method of modelling and neural network forecasting, various scenarios of the occurrence and development of an emergency at a tank farm in the Arctic territory of the Krasnoyarsk Territory was tested.
Accident scenarios of destruction of the onshore process pipeline in the area of possible impact on the lake, the area of the polluted water area depends on the lake into which oil products flow and may vary, depending on the area of the water surface of a particular lake located nearby, along the movement of the process pipeline.
As calculations show, when a spill of oil products is realized without fire and explosion, the spill cannot cause significant damage to health and property of the population and third-party organizations. Poisoning by oil vapours during combustion is possible for the facility personnel, as well as environmental damage from pollution by evaporated hydrocarbons from the surface of the oil spill.
The penetration of oil products into the water areas of stagnant bodies of water without further spread is predicted in the presence of an ice crust. In this case, the spilled oil products are poured onto the ice surface.
The penetration of oil products into the water area of the Yenisei River with further spread along its course is predicted only at a positive ambient temperature and subject to the presence of an open current in the streams (the stream is not frozen or not dried up).
When an emergency situation develops according to the scenario of an explosion of oil products, it is predicted that material damage will be caused to technological equipment and structures of other industrial sites.
When developing according to the scenario of an explosion of a fuel assembly or a combustion of a fuel assembly - "fire-flash", damage to the life and health of third-party organizations is possible.
The most dangerous scenario associated with a risk to human life and health is an accident with the release of oil products and the formation of a spill, and further formation and explosion.
In this work, we also compared the analytical and neural network methods for predicting the development of an emergency. The neural network was trained on the basis of real data obtained during an accident at a thermal power plant in Norilsk in 2020. In general, the results obtained indicate the applicability of the neural network forecasting method. In comparison with the analytical forecasting method, the neural network method allows relatively faster making the necessary calculations by means of automation and adaptation of the model for each potentially dangerous object and, in the event of an emergency, to make the necessary calculations to assess the situation and make a decision on attracting additional forces and means necessary for elimination accident.
The results of the work testify to the importance of the problem of technical supervision of technological equipment when used in the fragile ecosystem of the Arctic. The results of the work can be applied in the development of plans for the prevention and elimination of emergency spills of oil and oil products.
Acknowledgments
The study was carried out with the support of the Krasnoyarsk Regional Science Foundation within the framework of the project: Development of a set of necessary preventive measures to protect the population and the Arctic territory of the Krasnoyarsk Territory from natural and man-made emergencies based on a neural network assessment of the occurrence.
References
Cappuyns, V. (2013). Environmental impacts of soil remediation activities: quantitative and qualitative tools applied on three case studies. Journal of cleaner production, 52, 145-154.
Carlon, C., Critto, A., Ramieri, E., & Marcomini, A. (2007). DESYRE: Decision support system for the rehabilitation of contaminated megasites. Integrated Environmental Assessment and Management: An International Journal, 3(2), 211-222.
Chuchueva, I. A. (2010). Time series forecasting using a maximum likelihood extrapolation model. Science and modernity: collection of materials of the International Scientific and Practical Conference, 187-192.
Dorrer, G. A. (2008). Dynamics of forest fires. Novosibirsk: Publishing house of the SB RAS, p. 404.
Fentiman, A., & Zabbey, N. (2015). Environmental degradation and cultural erosion in Ogoniland: a case study of the oil spills in Bodo. The Extractive Industries and Society, 2(4), 615-624.
Gorban, A., Rossiev, A., Makarenko, N., Kuandykov, Y., & Dergachev, V. (2002). Recovering data gaps through neural network methods. International Journal of Geomagnetism and Aeronomy, 3(2), 191-197.
Gosgortekhnadzor of Russia. (2002). RD 13.020.00-KTN-148-11 Methodological guidelines for assessing the degree of risk of accidents on oil trunk pipelines and oil product pipelines. http://www.omegametall.ru/Index2/1/4293854/4293854459.htm
Grebnev, Ya., & Moskalev, A. (2020). Influence of hydroelectric power station on flood situation and modeling of hydrological characteristics. E3S Web Conf, 221, 1-7.
Grebnev, Ya., & Yarovoy, A. (2018). Monitoring and forecasting floods in the Krasnoyarsk Territory using neural network algorithms. Scientific and analytical journal "Siberian Fire and Rescue Bulletin", 3, 13-16.
Grebnev, Ya., Moskalev, A., Vershkov, A., & Gazizulina, A. (2019). The practice of connectionist model
Korovin, G. N. (1969). Methodology for calculating some parameters of ground forest fires. Collection of scientific research. forestry works. Proceedings of LenNIILH, XII(L.), 244-262.
Martyinov, O. V. (2011). Kontseptsiya sistemyi prognoza prirodnyih katastrof i prakticheskie rezultatyi poluchennyie na osnove apparatov nelineynoy fiziki [The concept of a system for forecasting natural disasters and practical results obtained on the basis of devices of nonlinear physics]. Nelineynyiy mir. [in Rus].
Medvedeva, O., & Solovieva, S. (2016). Methodology for the valuation of damage caused by atmospheric air pollution. Issues of valuation, 4(86), 2-6.
Mikheeva, A., & Ayusheeva, S. (2014). Comparative analysis of economic damage to the environment in the model areas. Bulletin of East Siberian State Uni of Tech and Man, 3(48), 92-96.
Moskvichev, V., Bychkov, I., Potapov, V., Taseiko, O., & Shokin, Y. (2017). Information system of territorial development and security risk management. Bulletin of RAS, 87(8), 696-705.
Moskvichev, V., & Nicheporchuk, V. (2018). Information support for monitoring and risks of socio-natural and man-made systems development. Problems of Risk Analysis, 15(2), 56-77.
NeuroPro. (2021). Neuroimmitator NeuroPro. http://www.neuropro.ru/
Potravny, I., & Ngo, T. (2018). Ensuring environmental safety of an enterprise: a constraint or a competitive advantage for business. Econ of Nat Res Man, 4, 89-102.
Rostekhnadzor. (2015). Order № 158 On approval of the Safety Guide: "Methodology for modeling the spread of accidental releases of hazardous substances". https://docs.cntd.ru/document/420271040
Toxi Industrial Safety Software. (2021). Automated environment modeling system Toxy + risk. https://toxi.ru/
Tsalikov, R. Kh. (2009a). Ministry of the Russian Federation for Civil Defense, Emergencies and Elimination of Consequences of Natural Disasters. Methodology for determining the calculated values of fire risk at production facilities. Moscow. https://docs.cntd.ru/document/902170886
Tsalikov, R. Kh. (2009b). Ministry of the Russian Federation for Civil Defense, Emergencies and Elimination of Consequences of Natural Disasters. Methods for determining the calculated values of fire risk at production facilities. https://docs.cntd.ru/document/902170886
Volchek, A. A. (2010). Neural network forecasting tools for flood control. Materials of the International Scientific and Practical Conference "Ensuring environmental safety - the path to sustainable development of Kazakhstan". Taraz: "Senim".
Copyright information
This work is licensed under a Creative Commons Attribution-NonCommercial-NoDerivatives 4.0 International License.
About this article
Publication Date
25 September 2021
Article Doi
eBook ISBN
978-1-80296-115-7
Publisher
European Publisher
Volume
116
Print ISBN (optional)
-
Edition Number
1st Edition
Pages
1-2895
Subjects
Economics, social trends, sustainability, modern society, behavioural sciences, education
Cite this article as:
Grebnev, Y. V., & Moskalev, A. K. (2021). Modeling The Assessment Of Technogenic Risks Impact At Arctic Enterprises. In I. V. Kovalev, A. A. Voroshilova, & A. S. Budagov (Eds.), Economic and Social Trends for Sustainability of Modern Society (ICEST-II 2021), vol 116. European Proceedings of Social and Behavioural Sciences (pp. 2641-2651). European Publisher. https://doi.org/10.15405/epsbs.2021.09.02.294