Abstract
The theoretical foundations of the VaR-method for the risk index calculation have been considered. The investigation applied monograph and analytical methods, artificial intelligence, design-constructive procedure, data quantization, “decision tree”, as well as analysis, modeling, study and generalization. The article deals with the AI neural network for predicting financial risk in the real economy of Russia. A hypothesis that the “decision tree” neural network enables obtaining a forecast of the extent of loss resulting from the financial risk has been put forward and proved. The relevance is due to the fact that in a “turbulent” economy, there is an increase in volatility in the parameters of the balanced profit of the Russian real economy, while the artificial intelligence systems applied to predict financial risk using the VaR method is important. The estimation of loss from financial risk caused by volatility especially matters in conditions of market uncertainty. The solution proposed is of great practical importance, since the AI-system developed provides support for managerial decision-making in terms of the balanced development of the real economy and financial sector.
Keywords: AI-systemforecastingfinancial riskVaR-modeleconomy digitalization
Introduction
The relevance of the research lies in the fact that the involvement of artificial intelligence in the risk evaluation and management is growing rapidly; however, certain aspects of the problems that arise in this case require further research. This article raises a problem that partially exists in science. The authors proposed an AI system processing BigData for predicting the financial risk in the real economy of Russia. A hypothesis that the neural network allows obtaining a forecast of the financial risk in Russia has been put forward and proved.
The solution proposed is of great practical importance, since the AI-system developed provides support for managerial decision-making in terms of the balanced development of the real economy and financial sector.
Of great importance is monitoring financial risk and investors’ inflation expectations, taking into account the dynamics of the VIX option—the “fear index” that reflects the investment activity level in the global economy.
All this, ultimately, allows ensuring financial stability and progressive development of the country's economy as a whole, in order to maximize the GDP of the country.
Evaluation of financial risk is important. There are many methods for evaluating and managing risk, but the artificial intelligence systems applied allow getting a breakthrough opportunity. So, the Russian Deductor platform (BaseGroup, Russia) makes it possible to use maximum potential of artificial intelligence systems. Of interest is the developed “decision tree” neural network that enables obtaining a forecast of financial risk in the real economy of Russia (Basegroup…, 2019).
The evaluation of the extent of loss resulting from financial risk caused by volatility is important in conditions of market uncertainty. In practice, the VAR-method risk calculation is widely used (VAR…, 2019).
The study has found many domestic and foreign works devoted to this problem. So, Lomakin et al. (2016) investigated issues of risk monitoring based on neural networks and fuzzy algorithms); Pavlov and Lomakin (2017) successfully conducted a study of the financial risks in organization by an artificial intelligence system.
Experience proved that the study of financial risks regarding the capitalization ratio of an enterprise in a particular field of activity is of great interest.
It seems appropriate to use the generally accepted risk evaluation technique. In essence, risk is a variance that is advisable to be represented as the standard deviation.
The standard deviation is determined by the formula (1)
,(1)
Where σ is the risk; n is the number of observations; ri is the profitability in the i-period; and ṝ is the average yield.
The spread of profitability with respect to the average value, and, consequently, the risk development can be compared.
The Value at Risk (VaR) method is based on the determination of the functional dependence of the risk on external factors and used for the quantitative risks evaluation in practice. The VaR-method is applied by the international Bank for International Settlements and others (Quantitative…, 2019).
The study proposed was faced with the fact that the category of “financial risk” is complex and multifaceted. The research showed that there are many works by domestic and foreign authors devoted to this problem. So, Lomakin et al. (2013) considered the exchange operations with company’s securities as a tool to reduce financial risks.
Many Russian and foreign scientists worked at the problem of reducing risk and increasing the sustainability of the Russian economy. For example, Baranov and Bessonov (2018) investigated the issues of economic transformation in Russia and the balance of the financial and economic system; studying the issues of profitable work of enterprises in the real economy in order to form a growth path for the future, Medvedev (2018) outlined the tasks of the economic policy of Russia.
In modern conditions, neural network models and artificial intelligence systems play an increasingly important role in solving the problems of evaluation and management of the financial risk, in particular, in financial forecasting (Koenecke & Gajewar, 2019). Curriculum learning in deep neural networks for financial forecasting. In Workshop on Mining Data for Financial Applications (pp. 16-31). Springer, Cham.2019), predicting sales in electronic commerce (Kui & Can, 2017), computer vision systems for automation of technological processes (Sakib et al., 2019), control in the interaction between humans and robots (Lathuilière et al., 2017), image recognition (Savchenko, 2017) and other areas.
Many areas, including the creation and promotion of the data science and analytics, are presented in the paper “Methods of Data Intellectual Analysis in Assessment of Economic Security” (Gontar et al., 2019). Very interesting are the brief studies on advanced information and knowledge processing presented by Jain et al. (2019). Today there are many known programs, a huge variety of different platforms and tools for analyzing big data. Here are some of them:
- frameworks (Hadoop, Spark and Storm);
- databases(Hive, Impala, Presto and Drill);
- analytical platforms (RapidMiner, IBM SPSS Modeler, KNIME, Qlik Analytics Platform, STATISTICA Data Miner, and Informatica Intelligent Data Platform); and
- other tools (Zookeeper, Flume, IBM Watson Analytics, Dell EMC Analytic Insights Module, Windows Azure HDInsight, Microsoft Azure Machine Learning and so on).
The Hadoop platform is an open-source framework that makes the application be divided into several fragments so that each of the obtained fragments is processed in any node (computer or “node”) of the cluster of the computing system developed (Lomakin et al., 2020).
BaseGroup Labs developed Deductor—an analytical platform, most popular analysis algorithms (decision trees, neural networks, self-organizing maps etc.) are embedded into; there are also dozens of visualization techniques and many data sources/receivers integration provided.
The Deductor platform allows
- retrieving data from different sources, consolidate the data in a single storage and display them in the form of reports and OLAP cubes;
- recognizing hidden patterns and evaluating the quality of models using DataMining; and
- segmentation of objects to be analyzed, identifying target markets and optimizing the work with consumers, for the resources being used more rationally.
Methods
Analysis, modeling, study and generalization
The investigation applied monograph and analytical methods, artificial intelligence, design-constructive procedure, data quantization, “decision tree”, as well as analysis, modeling, study and generalization.
In order to develop the model, we used statistical data reflecting the development of the economic sphere and the financial sector of Russia quarterly for the period from 2015 to 2018, as well as individual parameters of the global economy, including the US dollar and VIX-Index.
Taking into account the data on statistics and domestic financial system for the period of 2015-2018, a neural network model “decision tree” was formed. The following baseline data (fragment) were used in the model “Table
Based on the formula for calculating the variance of net profit of organizations in the real economy, the financial risk was calculated quarterly for the period under study “Table
The study found the key rate of the Central Bank to be an important factor that ensured the stabilization of financial risk arising from the volatility of net profit of organizations in the real sector of the economy “Figure
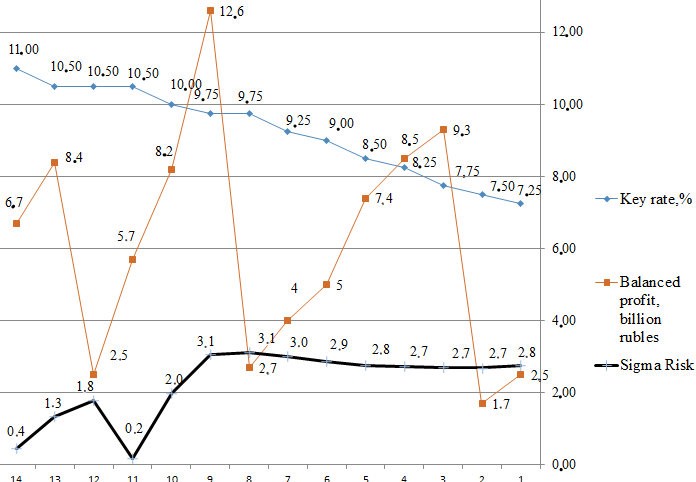
In turn, the key rate is a tool that regulated the effects of external factors, such as the oil price and ensured a balanced economic system in the face of market uncertainty “Figure
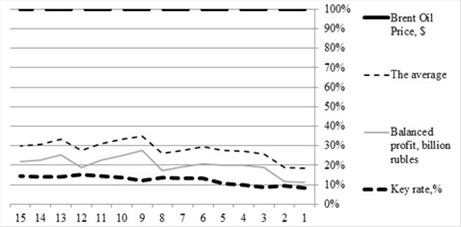
Thus, financial risk is an important factor underlying the balance of economic and financial sectors in order to achieve stable development of the country.
It seems appropriate to consider the dynamics of the financial risk parameters in more detail, using the VaR-method. Essential parameters, i.e. mathematical expectation, standard deviation, quantile and VaR were preliminary calculated in Excel “Figure
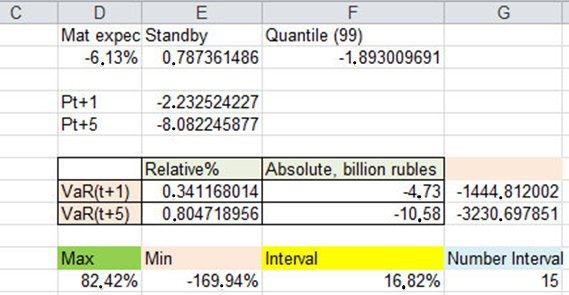
Based on the data obtained, a VaR-histogram of the financial risk resulting from the volatility of the consolidated profit of organizations in the real economy of Russian was calculated and Excel plotted “Figure
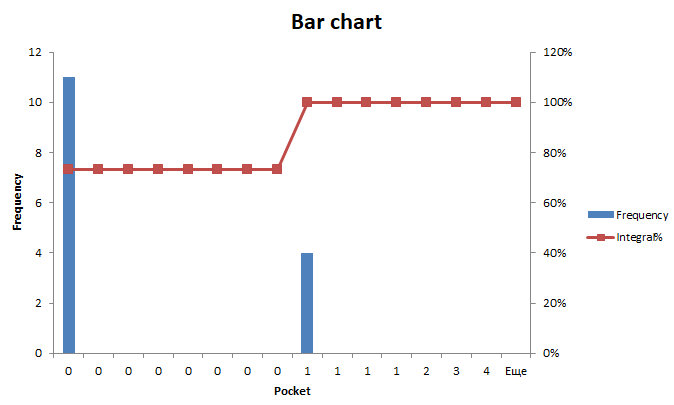
In order to develop a neural network model “decision tree” for predicting financial risk, it seemed appropriate to calculate the following parameters: Pt+1, Pt+5, VaR(t+1)%, VaR(t+1) blnRUB, VaR(t+5)%, VaR(t+5) blnRUB, quantile, mathematical expectation and standard deviation for each timeframe (Q) “Table
The data obtained were processed by the neural network using the data quantization method in the Deductor program “Table
The “Quantization” handler in the Deductor program allowed splitting the range of a numerical feature into a given number of intervals. Quantization can be used to reduce the dimensionality of data, namely, to reduce the number of various values of the factor (Quantization).
The structure of the tree consists of “leaves” and “branches”. The edges (“branches”) of the decision tree contain the attributes the objective function depends on, the values of the objective function are written in the “leaves,” and the attributes that distinguish cases are written in other nodes. To conduct a new classification, it is necessary to go down the tree to the leaf and issue the corresponding value. The decision trees of that kind are widely used in data mining because their goal is to create a model that predicts the value of the target variable based on several input variables “Figure
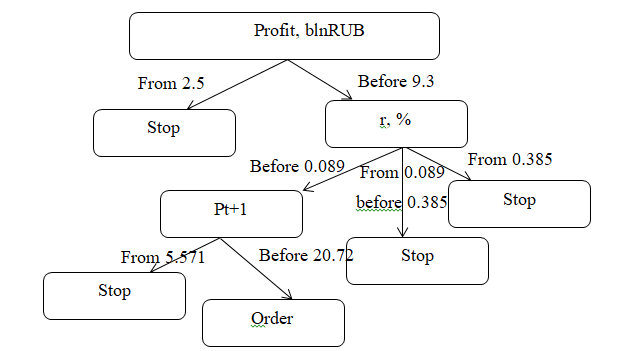
Processing the data of the neural network model in Deductor resulted in obtaining the “decision tree” program. A peculiarity differential of the neural network model obtained was that the range of values of the predicted financial risk VaR (t+1) blnRUB was divided into three intervals with the values of up to 3.0714, from 11.4257 and from 3.0714 to 11.4257 “Table
Using the “What-if” function in the Deductor program, it was not difficult to obtain the predicted values of the financial risk, with the model input parameters being applied “Figure
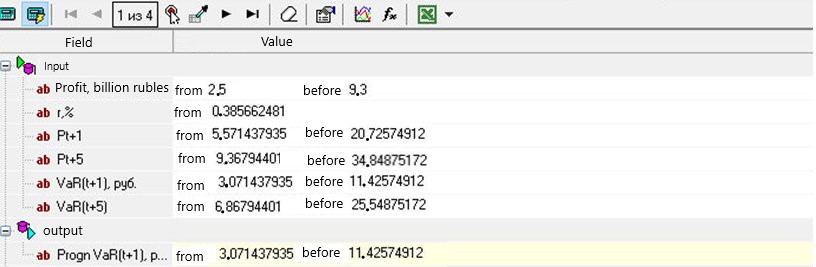
The forecast values of financial risk obtained ware summarized in “Table
The “decision tree” neural network allowed obtaining a wide range of predicted values of financial risk to support managerial decision-making in conditions of market uncertainty “Figure
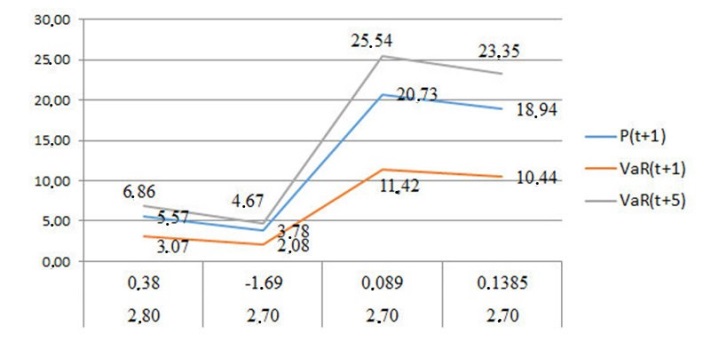
Results and discussion
A forecast of financial risk for organizations of Russian real economy
The aim of the study was to put forward and prove the hypothesis that the neural network makes it possible to develop a forecast of financial risk for organizations of Russian real economy. The theoretical foundations of the VaR-method for the risk index calculation have been considered.
The “decision tree” neural network
A hypothesis that the “decision tree” neural network enables obtaining a forecast of the extent of loss resulting from the financial risk has been put forward and proved. The relevance is due to the fact that in a “turbulent” economy, there is an increase in volatility in the parameters of the balanced profit of the Russian real economy, while the artificial intelligence systems applied to predict financial risk using the VaR method is important. The solution proposed is of great practical importance, since the AI-system developed provides support for managerial decision-making in terms of the balanced development of the real economy and financial sector.
Discussion
Value at Risk (VaR) method
The results of this study correlate with the experience of other scientists. Theoretical foundations of the Value at Risk (VaR) method based on the determination of the functional relationship between the risk probability and external factors were investigated. A hypothesis that the “decision tree” neural network enables obtaining a forecast of the extent of loss resulting from the financial risk has been put forward and proved.
The “decision tree” for predict financial risk
The “decision tree”is widely used to support managerial decision-making, in particular, to predict financial risk. The “decision tree” (also “classification tree” or “regression tree”) is a decision support tool used in machine learning, data analysis and statistics (Decision tree) and other (VAR calculation based on the GARCH model -1.1, 2019). Noteworthy is the study of Eason et al. (1955) regarding the product of Bessel functions.
Basic conceptual approaches were considered in order to reduce risk, ensure balance of the financial and economic system and increase the sustainability of the Russian economy, in particular, in the context of economic transformation.
The Main trends in risk management
Main trends in risk management of certain elements of the financial and economic system in the context of digitalization were identified. Main factors affecting the financial risk of organizations in the real economy of Russian conditions of market uncertainty were studied.
Main trends in the use of artificial intelligence systems in various fields of activity were studied. Profit dynamics of organizations in the Russian real economy was analyzed. The estimation of loss from financial risk caused by volatility especially matters in conditions of market uncertainty. The solution proposed is of great practical importance, since the AI-system developed provides support for managerial decision-making in terms of the balanced development of the real economy and financial sector.
Conclusions
Based on the study conducted, we can draw the following conclusions.
Firstly, the “decision tree” neural network allows visualization of data obtained from the VaR-analysis. Of great practical importance are the P (t+1), VAR (t+1) and VAR (t+5) parameters that characterize the value of actual financial risk and risk for the ensuing quarter (time frame).
Secondly, managing financial risk by a neural network is important.
Thirdly, the hypothesis proposed was proved, i.e., the “decision tree” neural network allows investigating and predicting the risk for the net profit of organizations in the real economy.
Fourth, the theoretical foundations for the artificial intelligence systems being applied in the processing of Big Data were investigated. A huge variety of different programs, platforms and tools available were considered with respect to the big data analysis and machine learning. A bench marking study of the capabilities of the Hadoop framework and the Deductor analytic platform was performed.
Fifth, for the growth of the digital economy, it is necessary to use more neural networks and stimulate the application of artificial intelligence systems to support decisions-making in the field of financial risk management. Greater government involvement should be achieved in the digitalization of the economy, so that information technologies are more actively implemented in all sectors.
Acknowledgements
The authors are grateful for the financial support provided by the RFBR grant No. 19-010-00985A Development of Innovation and Investment Policy as a Concept of Strategic Economic Security of Agricultural Organizations in the Conditions of Modern Technological Transformation.
References
- Baranov, E. F., & Bessonov, A. V. (2018). Vzglyad na rossijskuyu ehkonomicheskuyu transformaci [A look at Russian economic transformation]. Questions of economy, 11, 26-28.
- Basegroup Advanced analytics without programming. (2019, September 13). https://basegroup.ru/deductor/description
- Eason, G., Noble, B., & Sneddon, I. N. (1955). On certain integrals of Lipschitz-Hankel type involving products of Bessel functions. Philosophical Transactions of the Royal Society of London. Series A, Mathematical and Physical Sciences, 247(935), 529-551.
- Gontar, A. A., Lomakin, N. I., Gorbacheva, A. S., Chekrygina, T. A., & Tokareva, E. V. (2019). Methods of data intellectual analysis in assessment of economic security level. In Ubiquitous Computing and the Internet of Things: Prerequisites for the Development of ICT (pp. 455-464). Springer, Cham.
- Jain, L., Wu, X., Brahnam, S., Cook, D. J., Domingo-Ferrer, J., & Gabrys, B. (2009). Advanced information and knowledge processing. Springer.
- Koenecke, A., & Gajewar, A. (2019, September). Curriculum learning in deep neural networks for financial forecasting. In Workshop on Mining Data for Financial Applications (pp. 16-31). Springer, Cham.
- Kui, Z., & Can, W. (2017, August 26). E-commerce using Convolutional Neural Network https://z5h64q92x9.net/proxy_u/en-ru.ru/https/arxiv.org/abs/1708.07946v1
- Lathuilière, S., Massé, B., Mesejo, P., & Horaud, R. (2017, November 11). Neural Network Based Reinforcement Learning for Audio-Visual Gaze Control in Human-Robot Interaction, cs.RO | cs.CV | cs.NEPaper submitted to Pattern Recognition Letters. https://z5h64q92x9.net/proxy_u/en-ru.ru/https/arxiv.org/abs/1711.06834v2
- Lomakin, N. I., Korotina, V. A., Rasumnyj, A. S., & Biryukov, A. R. (2016). Financial risk management based on neural networks and fuzzy-algorithms. 15th scientific and practical conference of the teaching staff of VPI (branch) of Volgograd State Technical University: collection of abstracts. In 2 parts, Part 1. In S.I. Blaginina (Ed.). (pp. 225-227). VPI (branch) VSTU, Volgograd.
- Lomakin, N., Shokhnekh, A., Sazonov, S., Lukyanov, G., & Gorbunova, A. (2020). Hadoop and Deductor based digital AI system for predicting cost of innovative products in conditions of digitalization of economy. ACM International Conference Proceeding Series.
- Lomakin, N. I., Grishankin, A. I., & Maksimova, T. I. (2013). Exchange operations with securities of a company as a tool to reduce financial risks. Interaction between enterprises and universities – science, personnel and innovation: Proceedings of the IX interregional scientific-practical conf., (pp. 174-177). VPI (branch) VSTU. Volgograd, (CD-ROM).
- Medvedev, D. A. (2018). Rossiya-2024: Strategiya social'no-ehkonomicheskogo razvitiya [Russia-2024: Strategy for Socio-Economic Development]. Questions of economy, 11, 5-28.
- Pavlov, V. O., & Lomakin, N. I. (2017). The study of financial risks of organization by an artificial intelligence system. Step into the future: artificial intelligence and the digital economy: Proceedings of the 1st international scientific-practical conf. Is. 3. In P. V. Terelyansky, S. A. Lukyanova and E. N. Smirnova (Eds.), State University of Management.
- Quantitative risk assessment – the value at risk (VAR) method. (2019, September 9). http://www.beintrend.ru/value-at-risk-varvar
- Sakib, S., Ashrafi, Z., Siddique, Md., & Bakr, A. (2019 January 4). Implementation of Fruits Recognition Classifier using Convolutional, 04/23/2019. (v1: 4/1/2019) cs.CV / cs.LG. https://z5h64q92x9.net/proxy_u/en-ru.ru/https/arxiv.org/abs/1904.00783v3
- Savchenko, A. (2017, September 8). Functions in Image Recognition using Deep Learning Framework. https://z5h64q92x9.net/proxy_u/en-ru.ru/https/arxiv.org/abs/1708.02733v1
- VAR calculation based on the GARCH model (1.1). (2019). http://abnsecurities.blogspot.com/2018/02/var-garch11.html
Copyright information
This work is licensed under a Creative Commons Attribution-NonCommercial-NoDerivatives 4.0 International License.
About this article
Publication Date
08 March 2021
Article Doi
eBook ISBN
978-1-80296-102-7
Publisher
European Publisher
Volume
103
Print ISBN (optional)
-
Edition Number
1st Edition
Pages
1-644
Subjects
Digital economy, cybersecurity, entrepreneurship, business models, organizational behavior, entrepreneurial behavior, behavioral finance, personnel competencies
Cite this article as:
Lomakin, N., Maramygin, M., Sigidov, Y., Safonova, M., Kataev, A., & Maliy, N. (2021). Ai-System For Var-Method In Russia Economy In Conditions Of Modern Digital Transformation. In N. Lomakin (Ed.), Finance, Entrepreneurship and Technologies in Digital Economy, vol 103. European Proceedings of Social and Behavioural Sciences (pp. 326-336). European Publisher. https://doi.org/10.15405/epsbs.2021.03.41