Abstract
Traditional risk analysis and control measures are used to deal with hazard-based risks in ports. However, they are often incompetent in tackling threat-oriented risks which are of high uncertainty in data. This study proposes a novel method for risk analysis proposed using the hybrid of Bayesian Networks (BNs) and Root Cause Analysis (RCA), aiming to tackle the risk of terrorist attacks (TA)s in ports. In the post 9/11 era, TAs have been classified as an emerging risk that can disrupt any business, where it has a low likelihood but a high consequence. In seaports, TA patterns are varied where terrorists usually attack a port at its weak points (certain port facilities) which can cause a high impact of casualties. Therefore, it is important for port stakeholders to identify that weak point. In the model, different types of possible TAs are explored and identified with respect to various port sites and facilities through a literature review and historical accident analysis. The risk of each identified M-T pair is then evaluated using the BN-RCA model to prioritise their associated risk probabilities. In this process, historical data is used to identify and quantify the possible attack modes while subjective data is employed to analyse the risk probabilities of each pair of M-T. The study's findings may be applied as a stand-alone method for ranking essential systems, such as port facilities with high-risk or as part of a decision-making method for security control by calculating the consequence.
Keywords: Bayesian network, root cause analysis, maritime risk, terrorist attack, maritime security
Introduction
Acting as the critical node in international transportation, a seaport is the interface between sea and land and hence plays a critical role in ensuring the efficiency and seamless operation of a supply chain (SC) network that is becoming more complicated (Ng, 2007; Robinson, 2002; Yang et al., 2014). Disruptions in major ports could cause high economic loss and disorder of international SCs, revealing a high-risk stake of port operations. However, due to the engagement and interactions of multiple stakeholders, seaport risk control becomes more challenging (Brooks & Pelot, 2008). Numerous research on SC disruption risks in relation to new policy changes, new technologies, and applications of various information technology programmes have been done (e.g., Alyami et al., 2014; 2019). Natural and man-made calamities like floods, earthquakes, hurricanes, labour strikes, financial crises, or TAs are examples of disruptive risks (Abdul Halim, 2020; Kleindorfer & Saad, 2005).
Terrorism is the conscious use of illegal brutality or the use of criminal force as a threat to frighten governments or communities in order to compel or terrify them with political, religious, or ideological goals (United States Army Combined Arms Center, 2008). The 9/11 TAs triggered a new security dimension in almost all the business sectors including the port industry. Deducing a security risk pattern for anti-terrorism preventive measures is however difficult given that the traditional quantitative risk assessment (QRA) methods are not applicable in the cases that involve high uncertainty in risk data. Risk analysis using little objective data becomes necessary within the context of risk analysis of TAs. It goes without saying that taking emergency actions without any planning or defensive weaponry will be impossible when a TA arises and hits a port (Snyder & Tomlin, 2008).
To tackle this research challenge, this paper targets to upgrade a conceptual subjective security risk analysis method using the hybrid of Bayesian network (BN) and Root cause analysis (RCA) that enables port stakeholders 1) to identify different types of facilities that can be locked as an attack target by terrorists, 2) to analyse the types of attack modes (M) against the identified targets (T) in ports (i.e. pairs of M-T), and 3) to evaluate the risk perceptions for the port stakeholders on the security risk level of each pair of M-T.
This paper is set out in the following manner to fulfil the goal. The appropriate literature review is described in Section 2. The new security risk analysis model is created using BN and RCA in Section 3. Section 4 involves cases studies to demonstrate the feasibility of the developed model while Section 5 draws the conclusions with insightful research implications.
Literature Review
There are a few studies on port security that are associated with the International Ship and Port Facilities Security (ISPS) code (i.e., the most accepted regulation by the International Maritime Organisation (IMO) and used by all IMO member states’ ports in the world) (Alyami, 2014; 2019; Yang et al., 2014). They analyse the regulation, criticize it, and propose new ways in improving the regulation to counter maritime security risk. This rule was not just created with the idea of preventing terrorism in mind, but it was also created to combat robberies, piracy, thieveries and sabotage as a whole. The ISPS code strengthens the importance of security risk assessment but fails to provide a detailed quantitative methodology (Yang et al., 2014).
Risk definition and analysis in ports
Different forms of risk concepts are proposed in the risk sector, and each measures risk from different perspectives (Yu et al., 2020). Multiplying P and C is one of the most formal and well-established definitions of risk (R), where P is the probability of risk and C is the consequence (Rausand, 2013). However, in addition to the P and C, the risk is a complicated notion, and other variety of variables, such as uncertainty, exposure, and scenarios, are also included (Aven, 2012). For instance, Kaplan & Garrick (1981) add Specific Scenario (s), and Aven (2012) suggests considering Background Knowledge (BK), and Uncertainty of Data (U).
Within this context, it is suggested that R is to be measured through proof and observations following the Bayes risk theory. As opposed to the normal probabilistic theory, the Bayes risk theory models BK and U by using factors of earlier probability and conditional probability to illustrate a frequency probability and the interdependency among the risk components. As a result, risk should be updated to a model with a set of prior data and conditional probabilities as it is a dynamic notion that changes depending on the circumstances when new data is added to the probability model (Yu et al., 2020).
In fact, the port industry is a high-risk, high-return venture. The situation is made significantly worse by the emerging security risk of maritime terrorism toward ports. Due to its nature of randomness and scarcity in historical failure data, TAs are among the most unpredictable threats that can happen at both the sea and land sides of a port.
Terrorists who committed unlawful violence in a nautical environment, against ships or permanent platforms at sea or in ports, any passengers or crew aboard ships, and coastal infrastructure or centres involving resorts for tourists, port locations, and port cities are referred to as maritime terrorist (Bergqvist, 2014). In order to calculate risk posed by terrorists, NRC Committee has proposed a model from a terrorist perspective (Ezell et al., 2010). This model however needs to satisfy two important assumptions, which are 1) it assumes ideal adversary intelligence and rationality and 2) the intelligence community knows the objectives the adversary is trying to maximize in the first place. To complement the current state of the art in anti-terrorism risk studies, a new risk analysis model that can take into different stakeholder perspectives on the M-T pairs and their associated risk levels is highly demanded and beneficial.
Bayesian networks
BNs are visual models that combine probability theory with graph theory and it can compensate for the lack of secondary data or insufficient information, it is capable to mix several bits of information and employ expert judgement. An approach to solve sophisticated issues involving uncertainties in input data is provided by BN. BN also can combining simpler and smaller models, can formulate and present a complex system. BN can be used to make various prediction, diagnosis, and it can model the interdependencies among the root causes which cannot be achieved by simple hierarchical approaches such as fault tree analysis (Abdul Halim, 2020). Fault tree analysis reveals an either/or possibility which only presents the event that can occur or not (it is either one or zero probabilities). However, BN can model the partial occurrence of event B when event A occurs.
The probability of nodes, which is described as a means of communicating information or conviction that an event will occur or has occurred, is a characteristic of BN (Abdul Halim, 2020). The Bayesian model allows relevant information which acts as influencing factors to be incorporated. The deeper knowledge of the parameter failure rate that has resulted from the inclusion of these influencing factors, has increased trust in the analysis of the overall results. It is recommended that a BN model can be deployed using the prior information gathered for each influencing parameter together with the advice of experts (Jones et al., 2010). The methods make BN a trustworthy model for probabilistic inference by allowing the influence of evidence regarding one node, facilitating the propagation of other nodes in multiple-connected trees. Due to such advantages, BNs have been widely applied in maritime/oceanic risk analysis (Chang et al., 2020; Yang et al., 2018), but in port yet (e.g., John et al., 2016).
Root cause analysis (RCA)
RCA refers to a specific underlying cause that can be identified, fixed and prevent recurrences can be generated (Rooney & Heuvel, 2004). RCA is used for fault localization, fault isolation or alarm/event correlation. It is the method of inferring the set of faults that cause a given set of symptoms. If the root cause/fault are directly observable, this process could be trivial, in which case they are also symptoms. However, in complex systems this is not the usual case. When there were one or more factors may actually constitute the root cause(s) of the problem being researched, it is established practice to refer to the root cause in a singular form. In this study, the procedure of identifying BN nodes is carried out using RCA. This strategy explains how an attack event could occur in container terminals owing to ambiguous conditions while also seeking a technique that can be utilized to describe causality (Rooney & Heuvel, 2004).
In the model, different types of possible TAs are explored and identified with respect to different port sites and facilities through a thorough literature review and secondary data analysis. Additionally, the brainstorm technique is used for facilitating the discussion with experts for subjective risk data elicitation. The RCA method is used in this process to explain the M-T pair identification and the interdependent nodes toward operation field experts. The investigation and mitigation of risks should be done in an organised manner and structured approach, but there are issues with RCA, where many RCAs are performed improperly or insufficiently and do not yield helpful data. Organizations frequently address each RCA separately, rather than drawing conclusions as a whole (Wu et al., 2008). Therefore, in this paper, we propose a holistic BN-RCA approach to take advantages of both methods. Specifically, it allows RCA to aid the identification of all the root causes, and a BN method to model the interrelationship among the risk factors for the risk prioritisation of the identified M-T pairs.
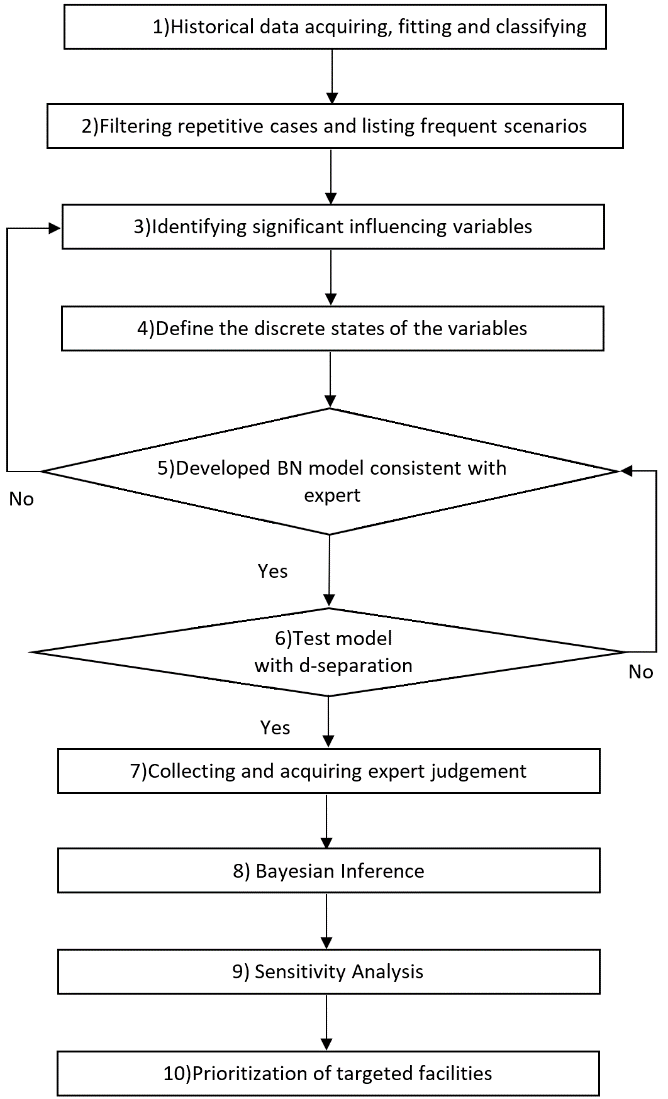
Methodology: Using of BN in assessing the Risk of TAs in Ports
BN is used in this section to identify port facilities that were vulnerable to ward TA and prioritize them i.e., M-T (Abdul Halim, 2020). The assessment process as shown in Figure 1, includes ten steps and some supportive approaches.
Step 1: Historical data acquiring, fitting and classifying
This step helps identify TA modes and targeted port sites/facilities (M-T) using historical data from Global Terrorist Database (GTD). This database shows a list of maritime TAs that cover the accidents at both sea and ports since 2001. After extracting port cases (including those in port waters), 55 cases were collected (see Appendix 1) and distributed in Figure 2 by years. It reveals that the overall tendency on terrorist accidents in ports is growing despite variation, which further illustrates the urgency and significance of this research work in practice.
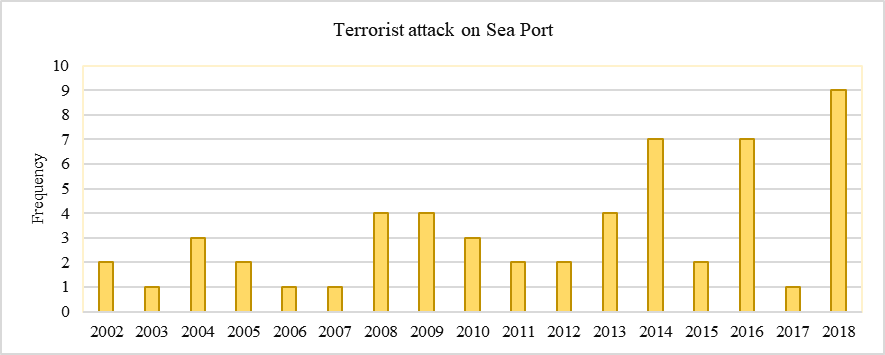
Step 2: Filtering repetitive cases and listing frequent scenarios
Based on this database, all 55 cases were analysed and filtered. Every repetitive case will be put together in a group and a list of frequent scenarios were generated. In order to confirm that the scenarios accurately reflect reality, expert opinions were sought. A selected expert denoted an individual with 5 to 20 years of involvement in the maritime security field. In this case, six experts were selected where three of them are from the port police department and another three from the rescue department. Specifically, a judgement involved weighing available proof and drawing a holistic conclusion. In the study context, an expert role provided the judgements based on the experiences involved in making sound evaluations.
Step 3: Identify the cause nodes
This step has been briefed extensively in Wan’s thesis which shows how researcher uses the RCA method to identify the cause node, verified it and classified it into primary causes and secondary causes (Abdul Halim, 2020). By doing so, the attacking modes (M) and the vulnerability port facilities (T) in the first two objectives can be met.
Step 4: Define the discrete states of the variables (Nodes)
Binary nodes are used in this initiating work in the field to simply the subjective data elicitation from domain experts (Wan, 2020). Therefore, two axioms were incorporated to be acceptable under the BN algorithm:
•For any event, 0 ≤ P (X=x) ≤ 1, with P (X=x) = 1 if and only if X=x happens with certainty.
•For any two mutually exclusive events X=x and X=y, the probability that either X=x or X=y occurs is P(X=x or X=y) = P(X=x) + P(X=y).
Step 5: Developing a BN Model
Confirming their relationships and building BN model based on their relationships (Abdul Halim, 2020).
Step 6: Check and modify the model by using a D-separation technique
Using D-separation technique to investigate the correctness of the network in this methodology (Wan, 2020).
Step 7: Data collection and analysis of each node
This paper uses both qualitative and quantitative data which can be into 3 steps mainly 1) collect data under the assumption, 2) qualitative data collected from experts 3) validation. Quantitative data is any data that can be counted or expressed numerically while addressing questions pertaining to quantity, frequency, value, or size such as the news report on the TA and the GTD that provided historical data on past maritime-oriented TAs. The qualitative and quantitative data are very different, but with both of them, a complete picture of an event can be captured. Data that resembles something or characterises an item or phenomenon is referred to as qualitative data. It is subjective, investigative, and focused on comprehension of a problem or situation and depends on descriptive words, images, and observations (Davidson, 2019) for example by interviewing several experts.
Collecting data under assumption
It was impossible to predict the behaviour of terrorists since they sought information and exploited weaknesses in defences to increase the impact of attacks (Abdul Halim, 2020). Therefore, to ensure consistent feedback from different experts, it is essential in an M-T scenario analysis, to set a condition that the terrorists have already set their T in an investigated port. As a result, in this paper, we assume an attack occurs by assigning a 100% prior probability across different root causes, and it will be helpful to prioritise the high-risk level M-Ts effectively.
Furthermore, setting the condition is to make sure there will be commonality in the scene for the experts and hence the received results from different experts are presented in a common plate and hence tend to consistent. It is simply because that the different setting leads to different interpretations of the TAs on a container port terminal. Furthermore, the data collection subject to an impression of imminent attacks facilitates the risk prioritisation.
Qualitative data calculation
This step has been briefed extensively in Wan’s thesis which shows how researchers calculate the qualitative data from experts by instructing them to fill up the range of scales in table 1 in responding for each of the situations given during the interview (Abdul Halim, 2020).
Those responses and answers collected from experts then were converted into a probability value and then averaged by following the formula below:
(1)
Validation
The model's output must at the very least conform to the following two axioms if the model is valid and its rationale is logical (Abdul Halim, 2020):
Axiom 1. A slight increment in the degrees of belief that an attack will happen should certainly result in the effect of a relative increment in the degrees of belief of the Risk of facilities being attacked.
Axiom 2. The total influence magnitudes of the combination of the probability variations from x attributes (evidence) on the values should be always greater than the one from the set of x – y (y ∈ x) attributes (sub-evidence).
Step 8: Bayesian Inference
This step has been briefed extensively in Wan’s thesis which shows how researcher uses Bayes’ Theorem in two ways 1) prior and 2) posterior and then use it in the NETICA Software tool (Abdul Halim, 2020).
Step 9: Sensitivity Analysis
This step has been briefed extensively in Wan’s thesis which shows how sensitivity analysis is important to prove the robustness of the BN model (Abdul Halim, 2020). Parameter sensitivity typically consists of a number of exams/tests in which to examine how a change in the parameter causes alters the model, the modeler sets several/different parameter values. By examining the uncertainties that are frequently connected to model parameters, sensitivity analysis also helps increase trust in the model (Breierova & Choudari, 2001). By doing so, the third objective of this study can be achieved, while insightful implications can be drawn appropriately.
Case study
Step 1: Historical Data Acquiring, Fitting and Classifying
This step has been briefed extensively in Wan’s thesis which shows how the researcher has acquired the historical data from Global Terrorism Database (Wan, 2020).
Step 2: Filtering Repetitive Cases and Listing Frequent Scenarios
Based on this database and the analysis of repetitive cases, four representative attack scenarios are generated. After the expert consultation about the four scenarios, they are verified as 1) terrorists to directly bomb the port, 2) to ramp the port gate or wharf, 3) to attack using weapons from outside and 4) internal attacks using forged employee identities. This also reflects in part, the findings from a previous maritime security study (i.e., Yang et al., 2009a), where the above numbers 1 and 4 scenarios were identified and analysed (Wan 2020).
Step 3: Identify Significant Influencing Risk Variables/Nodes
This step has been briefed extensively in Wan’s thesis which shows how the researcher sort out all the cases of terrorist attack that only happen in the port area, identify on how the whole sequence of attack happened and then list it as the terrorist attack mode on port (Abdul Halim, 2020): -
- Terrorists attacked the port using a truck filled with explosives.
- Terrorists hijacked a vessel and then use it to attack the port
- Terrorists smuggling an explosive in to the port compound
- Terrorists infiltrate the port as a worker
- Terrorists infiltrate the port as a visitor/outsider contractor
From that list, 19 nodes were derived which shows that the attacks may come from the sea and from the land and these nodes then were discussed and verified by the expert (Abdul Halim, 2020): -
1. Using Tampered Truck(s)
2. Hijack Using Vessel(s)
3. Overcome the Prevention of Unauthorized Entry
4. Suicide Collision by Trucks/Vessels
5. Using Tampered Containers
6. Overcome Identification of Employees
7. Overcome the Prevention of Unauthorized Document Access
8. Smuggling Unauthorized Containers (Bombs)
9. Overcome Routine Security Inspections
10. Container Bomb Attacks
11. Overcome Identification of Visitors
12. Overcome the Prevention of Unauthorized Introduction of Items into Port Facilities
13. Armed Attackers Overcoming the Prevention of Unauthorized Entry
14. Weapon Attacks
15. Port Gates
16. Wharf Operation Sites
17. Yard Operation Sites
18. Administration Sites
19. Security Level
Step 4: Define Discrete States of the Variables (Nodes)
This step has been briefed extensively in Wan’s thesis which shows how the researcher has assigned states on each node as shown in the Table 2 (Abdul Halim, 2020).
Step 5: Developing a BN model
A top-down approach was used in developing the model (See Figure 3) with the assistance of RCA in Step 3, starting with an attack mode (M), through an attack target/place (T) and ending at the goal node (SL) (Abdul Halim, 2020).
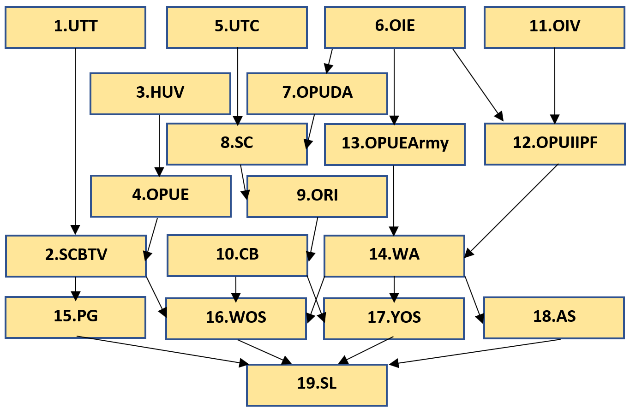
Step 6: Check and verify the model by using a d-separation technique
This step has been briefed extensively in Wan’s thesis which shows how the researcher has used d-separation technique to test the relationship of each node and a few modifications were made (Abdul Halim, 2020). Thus, Suicide Collision by Trucks/Vessels nodes was converted into two nodes, which is Suicide Collision by Trucks and Suicide Collision by Vessels
Step 7: Data collection and probability analysis of each node
Unconditional Probability
Five Unconditional Probabilities Tables (UCPTs) are obtained by using historical data from GTD. Among the 55 identified cases, there are 6 UTT related, 13 HVU, 15 UTC, 1 OIE, and 20 OIV. The unconditional probabilities of the give root nodes belonging to the state of “Yes” are calculated as 10.9%, 23.4%, 27.3%, 1.82% and 36.4%, accordingly, as seen in Table 3.
Results from the interviews and questionnaires
Six experts (from port security and maritime departments) undertook a survey. The data were then inserted in the Bayesian model created by using NETICA and then the result was generated. 15 Conditional Probabilities Tables (CPT) are obtained based on expert judgements and presented in Table 4.
The CPTs of the goal node referring to the security level are presented in Table 5, where the different given states are “Low Security” and “High Security”.
Step 8: Bayesian Inference
Using the Baye’s Theorem and the associated joint and marginal probability interference, the developed BN security model in Figure 4 together with the conditional probabilities analysed in Step 7, can be used to formulate a quantitative BN model for container terminal security level analysis. Here, a commercial software package Netica is used to facilitate the computation.
The results demonstrate that the posterior probability value of the node "WA-NO" increases from 68.8% (Figure 4) to 84.3% (Figure 5) after providing a piece of evidence to the node "OPUEARMY absolute NO" in Figure 7, hence the probability of “SL-High” increases from 82.8% (Figure 4) to 89.4% (Figure 5). It means that when WA (weapon attacks) becomes impossible in an investigated port, the port security level (belong to the state “High”) will increase.
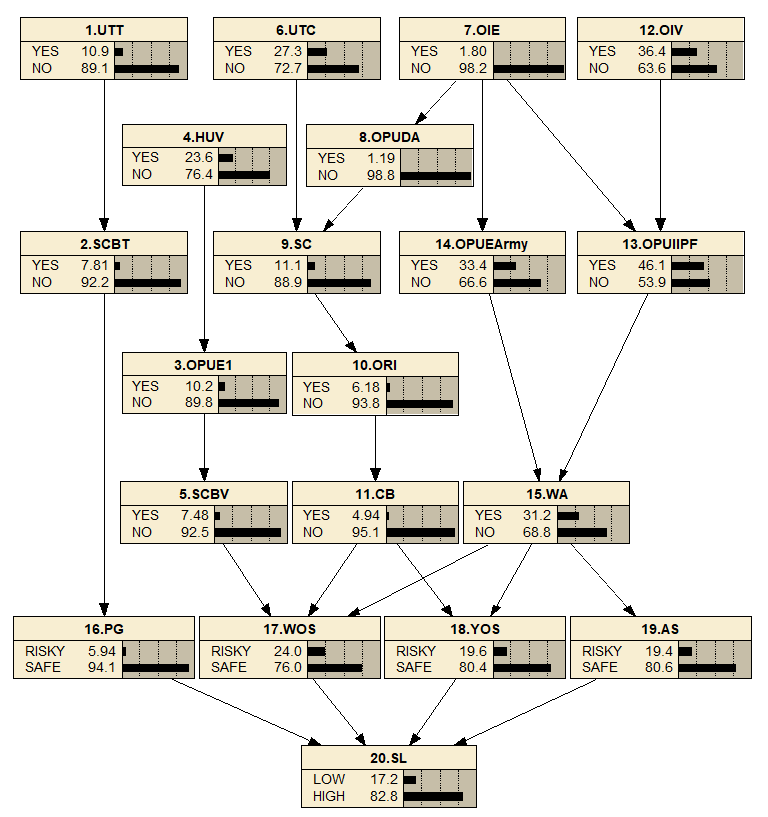
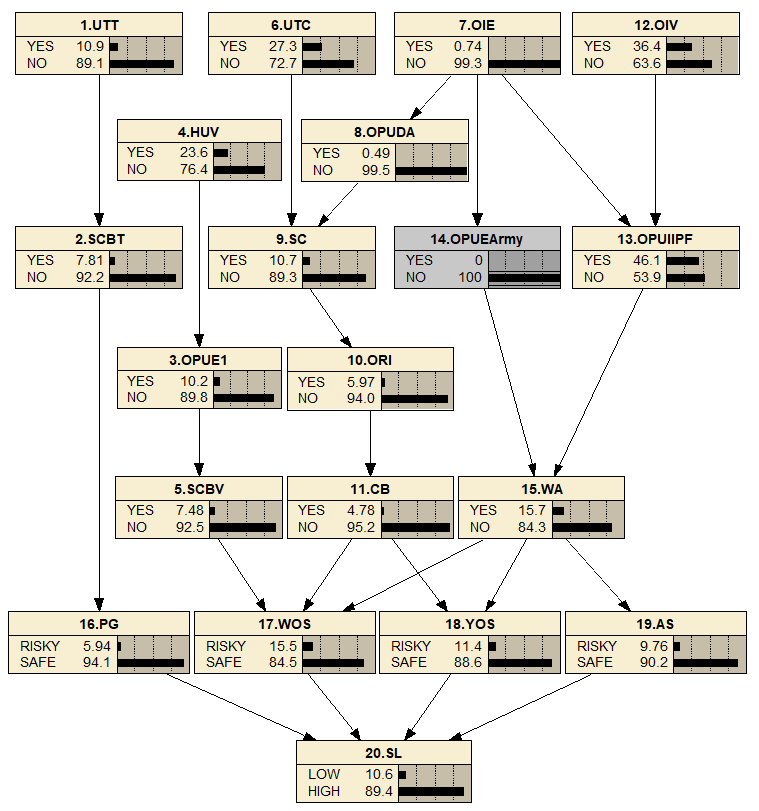
Step 9: Model Validation and Sensitivity Analysis
To guarantee its dependability, the produced BN is validated by a two-step validation process. The validation framework contains two conceptual tests of a face validity and content validity (Yu et al., 2020). The face validity tests the BN through a face investigation on the BN structures to improve the confidence of the developed relationships, whereas the content validity assesses the content of the BN can be accepted and consistent with reality (Pitchforth & Mengersen, 2013).
Face validity
By contrasting the correlations in the model with the expert's background knowledge and prior research, the face validity of the BN's rationality is validated. The expert panel introduced in Section 3 is invited to evaluate the model consistency. Not only do the elements utilised in the model, which cover every conceivable M-T pairs that affect TAs in ports, show excellent agreement with reality; the interactions between the factors also exhibit excellent consistency with the knowledge of the expert. It is an additional assurance to the model’s robustness beside the d-separation and RCA. As a result, the BN is recognised in the face validity and qualified to deliver accurate simulation on the TAs in seaports.
Content validity
The content validity tries to discuss if the BN's results are accurate. To lead to further risk mitigations, the most essential factor should be chosen. Plus, the importance of the selected factor should meet the human sense.To prioritise the variables, an entropy-based sensitivity analysis technique (for example mutual information analysis) is used. Here, we establish that a high entropy factor is more illuminating/informative than a low entropy factor. With the help of the Netica programme, the mutual information entropy for each node was determined, and the results are displayed in Table 6. It should be emphasised that the node of SL is chosen as the target to compare the relative importance between the target node and the related node.
The SL target node receives the greatest entropy value and variance among all the factors, with values of 0.65941 and 0.14159 respectively. With an entropy value of 0.18865 and relative relevance of 28.6%, the WA is determined to be the element that has the greatest impact on the SL. It followed by WOS, YOS and AS, which are three highly influential factors to the SL. The content validity shows the developed model follows Axiom 1 that was introduced in Section 3 (i.e. Step 7), thus validating the BN is rational and logical. To analyse the impact of each pair of M-T on the port security level, the results are obtained and presented in Table 7.
From Table 7, it reveals that the most influencing M-T pairs are Suicide Collisions by Trucks/Vessels in a decrement order, which obtains a severity level of 34.6%. The model shows two nodes (i.e. UTT and PG) are changed due to the potential influence from the incident. When the incident happens (i.e. trucks/vessels is 100% yes), the probability for using tempered trucks raise from 10.9% to 100% and the probability for port gates also significantly increase from 5.9% to 76%. It means that effective security risk control measures should be developed with respect to this priority list for the most effective risk reduction. In the meantime, it is noted the incident of smuggling unauthorized containers not only shows high risk (i.e. 26.9%) but also leads to wide fluctuations of seven nodes changes in the model. Comparing the abovementioned two M-T pairs, we find that some incidents like suicide collision can be defined as a typical direct incident, which has a short and simple causation chain but leads to high risk. In contrast, the causation chains for the incidents like smuggling unauthorised containers are more complicated, which has a wider range of influence and the state variations are slight and difficult to be detected. These indirect incidents are more easily ignored, that requires more attention in daily management. This finding can effectively help improve security risk control by focusing on direct incidents. In comparison with the results of single node evidence (i.e. x-y evidence in Axiom 2) the ones provide evidence that the created BN model is consistent with Axiom 2. Additionally, it confirms the model's resilience.
Conclusions
The developed BN-CRA model is dynamic and can be used to deal with the risk analysis of TAs in ports in different situations under uncertainty. In actual use, BN models allow port operators to include or discarded any node or parameter depending on the circumstances. Due to the models' adaptability in handling variable situations, they may be used in various uncertain conditions.
According to the case study's findings, port operators should focus their security efforts more on the weapon attacks because it has the most risks and is most likely to be the attacking approach (M), and for the target (T), the wharf operation site and the yard operation site; hijacking vessels has the least risk as compared to other attacking modes. This study has produced new contributions including 1) the consideration of the attacking risk from the view of the perpetrators (terrorists), 2) the identification of the most vulnerable facilities facing TAs, 3) the creation of a new risk model based on both historical data and subjective judgements to prioritise the risk levels of M-T pairs in a quantitative way.
Despite the above contributions, the current study has still revealed some limitations. The first limitation is the subjective data are collected from six experts. Although their judgements show good consistency, which helps on the confidence on data reliability. It is believed that more data from a large number of stakeholders could be collected and used to 1) generalise the findings and 2) critically analyse the security risk perceptions from different stakeholder groups to help rationalise the development of cost benefit control measures which can be easily accepted in practice. The other limitation is that given it is impossible to foresee the behaviour of TAs, to ensure a consistent feedback from different experts, a condition is set in which an attack attempt is confirmed as indicated by the sum of the five root cause node probabilities belonging to 100%. In future, more data should be collected to optimise the real occurrence probabilities of the five root nodes to provide a more realistic result that is not constrained by the set condition in this paper.
References
Abdul Halim, W. M. Z. (2020). Risk Assessment and Decision Making of Security in Container Port Facilities. Liverpool John Moores University (United Kingdom).
Acciaro, M., & Serra, P. (2013). Maritime SC security: a critical review. IFSPA 2013, trade SC activities and transport: Contemporary logistics and maritime issues, 636.
Ahokas, J., Kiiski, T., Malmsten, J., & Ojala, L. M. (2017). Cybersecurity in ports: a conceptual approach. In Digitalization in SC Management and Logistics: Smart and Digital Solutions for an Industry 4.0 Environment. Proceedings of the Hamburg International Conference of Logistics (HICL), Vol. 23 (pp. 343-359). epubli GmbH.
Alyami, H., Lee, P. T. W., Yang, Z., Riahi, R., Bonsall, S., & Wang, J. (2014). An advanced risk analysis approach for container port safety evaluation. Maritime Policy & Management, 41(7), 634-650. DOI:
Alyami, H., Yang, Z., Riahi, R., Bonsall, S., & Wang, J. (2019). Advanced uncertainty modelling for container port risk analysis. Accident Analysis & Prevention, 123, 411-421. DOI:
Aven, T. (2012). Foundations of Risk Analysis. John Wiley & Sons. DOI:
Bergqvist, L. (2014, March 28). The ISPS-Code and Maritime Terrorism. Center for International Maritime Security. http://cimsec.org/isps-code-maritime-terrorism/12098
Bhattacharya, A., Geraghty, J., Young, P., & Byrne, P. J. (2013). Design of a resilient shock absorber for disrupted SC networks: a shock-dampening fortification framework for mitigating excursion events. Production Planning & Control, 24(8-9), 721-742. DOI:
Bradford, J. F. (2004). Japanese Anti‑ Piracy Initiatives in Southeast Asia: Policy Formulation and the Coastal State Responses. Contemporary Southeast Asia, 26(3), 480–505. DOI:
Breierova, L., & Choudari, M. (2001). An introduction to sensitivity analysis. The Massachusetts Institute of Technology, 41–106. https://ocw.mit.edu/courses/sloan-school-of-management/15-988-system-dynamics-self-study-fall-1998-spring-1999/readings/sensitivityanalysis.pdf
Brooks, M. R., & Pelot, R. (2008) Port Security: A Risk-Based Perspective. In W. K. Talley (Ed.), Maritime Safety, Security and Piracy (pp. 195–216). LLP.
Brown, K. E. (2011). Muriel’s wedding: News media representations of Europe’s first female suicide terrorist. European Journal of Cultural Studies, 14(6), 705–726. DOI:
Chang, C. C., Kontovas, C., Qing, Y., & Yang Z. (2020). Risk assessment of the operations of maritime autonomous surface ships. Reliability Engineering and System Safety, 207, 107324. DOI:
Davidson L. (2019). “Quantitative Data: A Comprehensive Overview”, Available from (June 18, 2019) (https://www.springboard.com/blog/quantitative-data/)Davidson, L. (2020, July 8). Quantitative Data: A Comprehensive Overview. Springboard Blog. https://www.springboard.com/blog/quantitative-data/
Dwibhashyam, P. (2016, December 11). 16 killed in car bomb blast near port in Somalia capital Mogadishu. International Business Times UK. https://www.ibtimes.co.uk/three-killed-car-bomb-blast-near-port-somalias-capital-mogadishu-1595902
Eichensehr, K. (2009). Treason in the Age of Terrorism: An Explanation and Evaluation of Treason’s Return in Democratic States. Vanderbilt Journal of Transnational Law, 42(5), 1–65. https://ssrn.com/abstract=1634655
Ezell, B. C., Bennett, S. P., von Winterfeldt, D., Sokolowski, J., & Collins, A. J. (2010). Probabilistic Risk Analysis and Terrorism Risk. Risk Analysis, 30(4), 575–589. DOI:
GTD Maritime Incidents. (2018). Global Terrorism Database. https://www.start.umd.edu/gtd/search/Results.aspx?search=maritime&sa.x=40&sa.y=7
Guled, A. (2010, September 11). Somali Troops Foil Seaport Suicide Attack-Police. Reuters. http://www.reuters.com/article/2010/09/12/ozatp-somalia-conflict-idAFJOE68B01B20100912
Ho, M. W., & Ho, K. H. D. (2006). Risk Management in Large Physical Infrastructure Investments: The Context of Seaport Infrastructure Development and Investment. Maritime economics & logistics, 8(2), 140-168. DOI:
John, A., Yang, Z., Riahi, R., & Wang, J. (2016), A risk assessment approach to improve the resilience of a seaport system using Bayesian networks. Ocean Engineering, 111, 136-147. DOI:
Jones, B., Jenkinson, I., Yang, Z., & Wang, J. (2010). The use of Bayesian network modelling for maintenance planning in a manufacturing industry. Reliability Engineering & System Safety, 95(3), 267-277. DOI:
Jordan, M. I. (Ed.). (1998). Learning in graphical models (Vol. 89). Springer Science & Business Media. DOI:
Kaplan, S., & Garrick, B. J. (1981). On the quantitative definition of risk. Risk Analysis, 1(1), 11-27. DOI:
Khan, F. (2016, May 31). A Chinese Engineer were wounded from a blast in Karachi. Tribune. https://tribune.com.pk/story/1113261/chinese-engineer-wounded-roadside-blast
Kleindorfer, P. R., & Saad, G. H. (2005). Managing disruption risks in SCs. Production and Operations Management, 14(1), 53-68. DOI:
Korb, K. B., & Nicholson, A. E. (2003). Bayesian artificial intelligence. Chapman & Hall/CRC Press. DOI:
Kurapati, S., Lukosch, H., Verbraeck, A., & Brazier, F. M. (2015). Improving resilience in intermodal transport operations in seaports: a gaming approach. EURO Journal on Decision Processes, 3(3), 375-396. DOI:
Loh, H. S., & Thai, V. V. (2015). Management of Disruptions by Seaports: Preliminary Findings. Asia Pacific Journal of Marketing and Logistics, 27(1), 146-162. DOI:
Marsh & McLennan Companies. (2014). “Ports and Terminals Risk Challenges and Solutions” Global Infrastructure and Marine Practices. Published 7/2014. https://www.oliverwyman.com/content/dam/ marsh/Documents/PDF/US-en/Ports%20and%20Terminals%20Risk%20Challenges%20and%20 Solutions-06-2014.pdf
Mroszczyk, J. (2019). To die or to kill? An analysis of suicide attack lethality. Terrorism and Political Violence, 31(2), 346-366. DOI:
Neapolitan, R. E. (1990). Probabilistic Reasoning in Expert System: Theory and Algorithms. John Willey Sons, Inc.
Ng, A. K. Y. (2007). ‘Port Security and the Competitiveness of Short Sea Shipping in Europe: Implications and Challenges. In K. Bichou, M. Bell, & A. Evans (Eds.), Risk Management in Port Operations, Logistics and SC Security (Chapter 20, pp. 347-366). LLP.
Jensen, F. V., & Nielsen, T. D. (2007). Bayesian networks and decision graphs. Springer Science & Business Media. DOI:
Okwesili, P., Mazzuchi, T., & Sarkani, S. (2016). Risk assessment using paired comparison expert judgment for ranking of compounding outsourcing facilities. IEEE Engineering Management Review, 44(1), 47-56. DOI:
Pareño, R. (2016, February 26). Blast hits Basilan port terminal. Philstar.Com. https://www.philstar.com/nation/2016/01/19/1544272/blast-hits-basilan-port-terminal
Pearl, J. (1986). Fusion, propagation and structuring in belief networks. Artificial Intelligence, 29(3), 241-288. DOI:
Pearl, J. (1988). Probabilistic reasoning in intelligent systems: networks of plausible inference. Morgan Kaufmann Publishers. DOI:
Pinto, C. A., & Talley, W. K. (2006). The Security Incident Cycle of Ports. Maritime Economics & Logistics, 8(3), 267-286. DOI:
Pitchforth, J., & Mengersen, K. (2013). A proposed validation framework for expert elicited Bayesian Networks. Expert Systems with Applications, 40(1), 162-167. DOI:
Rahman, N. A. (2012). Selection of the most beneficial shipping business strategy for containerships. European Journal of Business and Management, 4(17), 153-167.
Raman, B., & Hyderabad, C. (2010, June 20). Why LTTE Attacked Galle Naval Base and Harbour? International Terrorism Monitor-Paper No. 141. https://web.archive.org/web/20100620201219/http://southasiaanalysis.org/papers20/paper1997.html
Rausand, M. (2013). Risk assessment: theory, methods, and applications (Vol. 115). John Wiley & Sons. DOI:
Robinson, R. (2002). Ports as elements in value-driven chain systems: the new paradigm. Maritime Policy & Management, 29(3), 241-255. DOI:
Rooney, J. J., & Heuvel, L. N. V. (2004, July). Quality Basics: Root Cause Analysis for Beginners. American Society for Quality. https://www.academia.edu/32930385/Root_Cause_Analysis_ For_Beginners
Shachter, R. D. (1998). Bayes-ball: The Rational Pastime (For Determining Irrelevance and Requisite Information in Belief Networks and Influence Diagrams). Proceedings of the 14th Conference on Uncertainty in Artificial Intelligence (pp. 480-487).
Snyder, L. V., & Tomlin, B. (2008). Inventory management with advanced warning of disruptions. PC Rossin College of Engineering and Applied Sciences, Lehigh University, Bethlehem.
United States Army Combined Arms Center. (2008, September 17). Terrorism. http://usacac.army.mil/cac2/call/thesaurus/toc.asp?id=29533
Wang, J., & Trbojevic, V. (2007). Design for Safety of Large Marine and Offshore Engineering Products. Institute of Marine Engineering, Science and Technology.
Wu, A. W., Lipshutz, A. K., & Pronovost, P. J. (2008). Effectiveness and efficiency of root cause analysis in medicine. Jama, 299(6), 685-687. DOI:
Xinhua (2008, June 2). Indonesian bomb disposal unit blows suspicious package. People’s Daily Online. http://en.people.cn/90001/90777/90851/6422816.html
Yang Z., Bonsall S., Wang J., Fang Q. G., & Yang J. B. (2005). Subjective risk assessment of container SCs. International Journal of Automation and Computing, 2(1), 20-28. DOI:
Yang, Z., Bonsall, S., & Wang, J. (2009a). Use of fuzzy evidential reasoning in maritime security assessment. Risk Analysis, 29(1), 95-120. DOI:
Yang Z., Bonsall S., & Wang J. (2009b). Use of hybrid multiple uncertain attribute decision making techniques in safety management. Expert Systems with Applications, 36(2), 1569-1586. DOI:
Yang, Z., Ng, A., Lee, P. T. W., Wang, T., Qu, Z., Rodrigues, V. S., Pettit, S., Harris, I., Zhang, D., & Lau, Y. T. (2018). Risk and cost evaluation of port adaptation measures to climate change impacts. Transportation Research Part D: Transport Environment, 61, 444-458. DOI:
Yang, Z., Ng, A. K., & Wang, J. (2014). A new risk quantification approach in port facility security assessment. Transportation research part A: Policy and Practice, 59, 72-90. DOI:
Yu, Q., Liu, K., Chang, C. H., & Yang, Z. (2020). Realising advanced risk assessment of vessel traffic flows near offshore wind farms. Reliability Engineering & System Safety, 203, 107086. DOI:
Zhang, D., Yan, X., Yang, Z., Wall, A., & Wang, J. (2013). Incorporation of formal safety assessment and Bayesian network in navigational risk estimation of Yangtze River. Reliability Engineering & System Safety, 118, 93-115. DOI:
Copyright information
This work is licensed under a Creative Commons Attribution-NonCommercial-NoDerivatives 4.0 International License.
About this article
Publication Date
18 August 2023
Article Doi
eBook ISBN
978-1-80296-963-4
Publisher
European Publisher
Volume
1
Print ISBN (optional)
-
Edition Number
1st Edition
Pages
1-1050
Subjects
Multi-disciplinary, Accounting, Finance, Economics, Business Management, Marketing, Entrepreneurship, Social Studies
Cite this article as:
Zulhilmi, W. M., Yu, Q., Mokhtar, K., Said, H., & Yang, Z. (2023). Risk Analysis of Terrorist Attacks on Seaport Facilities Using Bayesian Networks. In A. H. Jaaffar, S. Buniamin, N. R. A. Rahman, N. S. Othman, N. Mohammad, S. Kasavan, N. E. A. B. Mohamad, Z. M. Saad, F. A. Ghani, & N. I. N. Redzuan (Eds.), Accelerating Transformation towards Sustainable and Resilient Business: Lessons Learned from the COVID-19 Crisis, vol 1. European Proceedings of Finance and Economics (pp. 419-438). European Publisher. https://doi.org/10.15405/epfe.23081.36